As we move beyond automation and into augmentation, AI is evolving from task-doer to decision-maker – from detecting diabetic retinopathy to deploying drones that sense wildfires ahead of time yet one principle stands firm: technology is only as precise as the human expertise guiding it; AI systems don’t learn autonomously. They require structured human input, domain-informed decisions, and rigorous validation loops to function reliably in the real world.
Even the most advanced models rely on human feedback in AI to interpret nuance, resolve ambiguity, and handle exceptions. Traditionally, this has taken the form of Human-in-the-Loop (HITL) systems, where human annotators or reviewers assist AI model training. HITL has been especially effective in scaling AI by leveraging generalist annotators for tasks like image labeling, Text labeling, language correction, content filtering, etc.
However, as AI expands into high-precision AI, contextual depth, high-stakes, and high-context environments, the expectations around accuracy, fairness, and accountability have risen dramatically. Whether it’s diagnosing tumors, moderating borderline content, misinterpreting legal disclosures, or generating human-like responses—the cost of “getting it wrong” is no longer negligible.
This brings us to a critical evolution in the AI lifecycle: From Human-in-the-Loop to Expert-in-the-Loop (EITL).
Unlike traditional HITL models that rely on general human input, EITL systems ensure it’s not just any human giving feedback—it’s the right human – a subject matter expert, a domain insider, a specialist whose input doesn’t just fine-tune a model but defines its ability to understand complex environments. “Expert-in-the-loop” (EITL) refers to a hybrid approach in artificial intelligence where human expertise is integrated into the machine learning process. This methodology involves combining the capabilities of machine learning algorithms with human domain knowledge or judgment to improve the accuracy, efficiency, and interpretability of AI systems.
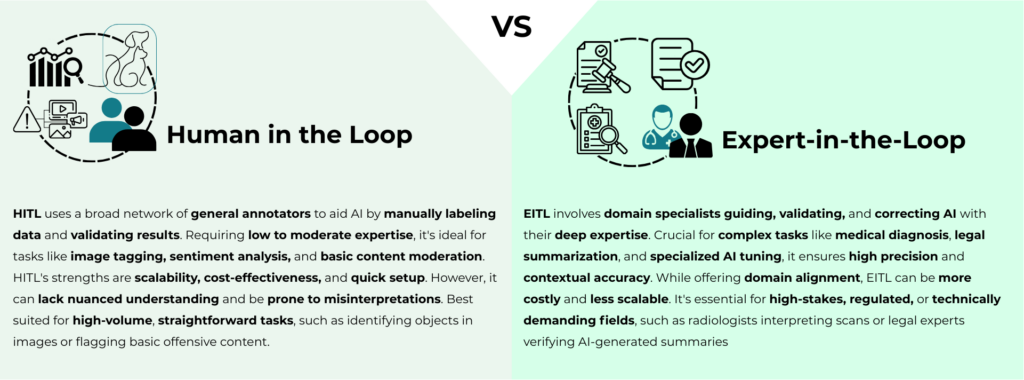
Why does this evolution matter now?
AI today is not just about scaling—it’s about AI accuracy improvement, compliance, and ethical AI development. As organizations integrate AI into critical sectors, systems built with expert guidance emerge as more robust and deployment ready. HITL vs EITL is no longer a debate—it’s a design choice that defines outcomes. It’s how we move from “good enough” models to “deployment-ready” systems.
Real-World Use Cases of Expert-in-the-Loop (EITL)
The move from Human-in-the-Loop (HITL) to Expert-in-the-Loop (EITL) isn’t just theoretical—it’s already shaping high-stakes AI workflows across industries. Here’s how:
- Healthcare – Radiologists step in to label complex anomalies in scans, training AI models to detect early-stage diseases.
- LegalTech – AI can draft summaries, but legal experts still review contracts for nuance and compliance.
- Automotive – Self-driving cars, early bug detection through simulated testing helps engineers fix issues like confusing a pedestrian with a window reflection or handling erratic movement, before integration into self-driving systems.
In all these cases, AI doesn’t replace expertise—it amplifies it.
Challenges in Implementing Expert-in-the-Loop (EITL)
The transition to Expert-in-the-Loop (EITL) represents a crucial leap toward building more accurate, explainable, and trustworthy AI systems. But this evolution doesn’t come without its share of structural and operational challenges.
- Scalability vs. Specialization – Unlike Human-in-the-Loop (HITL) models that scale efficiently through general annotators, EITL relies on specialized domain knowledge. This creates a fundamental tension—how do we scale AI training while still embedding deep domain expertise in areas like healthcare, law, etc.?
- Cost of precision – High-quality feedback from subject matter experts in AI increases operational costs. However, this cost must be weighed against the long-term value of AI accuracy improvement in mission-critical systems—where mistakes are expensive, both financially and reputationally.
- Tools & Integration – Successful EITL implementation hinges on robust tooling: expert annotation platforms, feedback orchestration systems, and synchronous collaboration environments—most of which aren’t readily available.
Despite these challenges, EITL is becoming a critical enabler of high-precision AI—especially where trust, compliance, and accountability are non-negotiable.
The Future of EITL in AI Development
- Fuelling Continual Learning – AI doesn’t stop learning after deployment. With Expert-in-the-Loop (EITL), models can be continuously refined using real-world feedback from domain experts—leading to smarter, more adaptive systems.
- HITL + EITL Hybrid Frameworks – The future lies in combining scale and depth.
- Human-in-the-Loop (HITL) = scalable data labeling
- Expert-in-the-Loop (EITL) = high-precision validation
Together, they create balanced, cost-effective AI pipelines.
- Essential for Regulated Domains – In industries like healthcare, finance, and legal, compliance and accuracy are non-negotiable. EITL ensures AI outputs meet regulatory standards while minimizing risk and bias.
Wrapping It Up: From HITL to EITL — A Shift That Matters
AI has come a long way thanks to Human-in-the-Loop (HITL) methods. But as we push into more complex, high-risk domains—healthcare, finance, legal, Gen AI—it’s no longer just about volume or quantity, it’s about precision, trust, and expertise.
That’s where Expert-in-the-Loop (EITL) steps in. It’s not just a fancy upgrade—it’s a mindset shift. Involving domain experts helps build AI that’s not only smarter but also safer, unbiased, and more aligned with real-world complexity.
Final Thought
AI will shape the future—but experts will shape AI.
Why Leading AI Teams Choose NextWealth
At NextWealth, we combine the scale of Human-in-the-Loop (HITL) with the precision of Expert-in-the-Loop (EITL) to deliver high-precision AI solutions across domains like Catalog, Healthcare, Gen AI, and Trust & Safety.
By embedding subject matter experts in AI model training, we enhance data annotation, ensure robust AI model validation, and drive consistent AI accuracy improvement—all while aligning with the goals of ethical AI development.
If you’re building AI that must perform in the real world, NextWealth is your partner in the loop